Securing the AI Transformation
Achieve AI security and safety to unblock the enterprise AI mission.
Get a DemoTrusted by enterprises worldwide
Securing AI in enterprises is hard
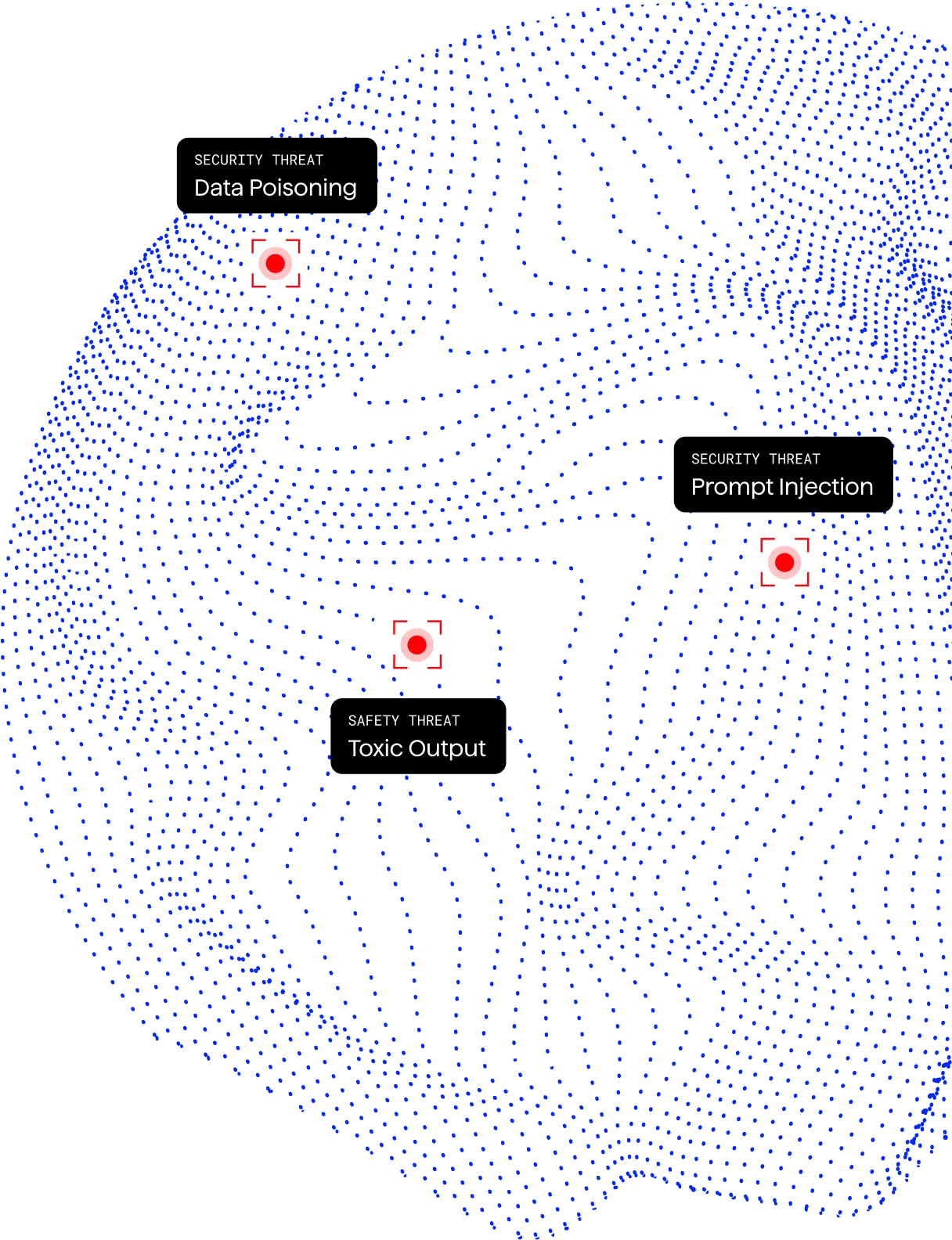
End-to-end security for AI applications
Robust Intelligence protects enterprises from AI security and safety vulnerabilities using an automated approach to assess and mitigate threats. The Robust Intelligence platform consists of two complementary components, which can be used independently but are best when paired together:
AI Validation
Automate evaluation of AI models, data, and files for security and safety vulnerabilities and determine required guardrails for secure AI deployment in production.